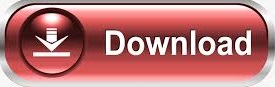
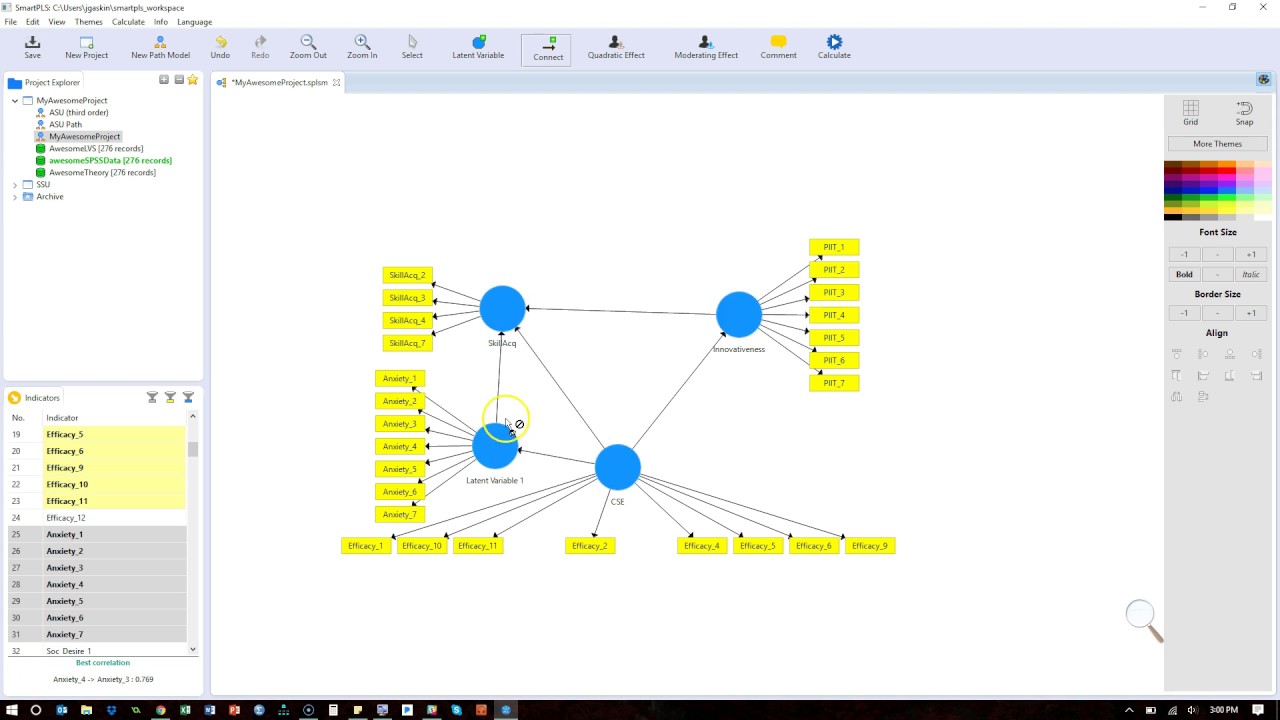
In particular, we create a graphical representation of the necessary steps to take and decisions to make in the form of a flow chart. How can the significance of moderating effects be determined?īorrowing from the body of knowledge on modeling interaction effect within multiple regression, we develop a guideline on how to test moderating effects in PLS path models. How can the coefficients of moderating effects be estimated and interpreted?And, finally: How does the type of measurement model of the independent and the moderator variables influence the detection of moderating effects?īefore the model estimation, should the data be prepared in a particular manner? Should the indicators be centered (by having a mean of zero), standardized (by having a mean of zero and a standard deviation of one), or manipulated in any other way? How can a moderating effect be drawn in a PLS path model, taking into account that the available software only permits direct effects?

We provide profound answers to typical questions related to testing moderating effects within PLS path models: comparisons of model estimates for different groups of observations, represent a special case of moderating effects by having the grouping variable as a categorical moderator variable. We also show that group comparisons, i.e. We illustrate the identification and quantification of moderating effects in complex causal structures by means of Partial Least Squares Path Modeling. Investigators using partial least squares path modeling need appropriate means to test their models for such moderating effects. Moderating effects are evoked by variables whose variation influences the strength or the direction of a relationship between an exogenous and an endogenous variable. Besides the examination of direct effects, researchers are more and more interested in moderating effects. Along with the development of scientific disciplines, namely social sciences, hypothesized relationships become increasingly more complex.
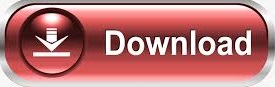